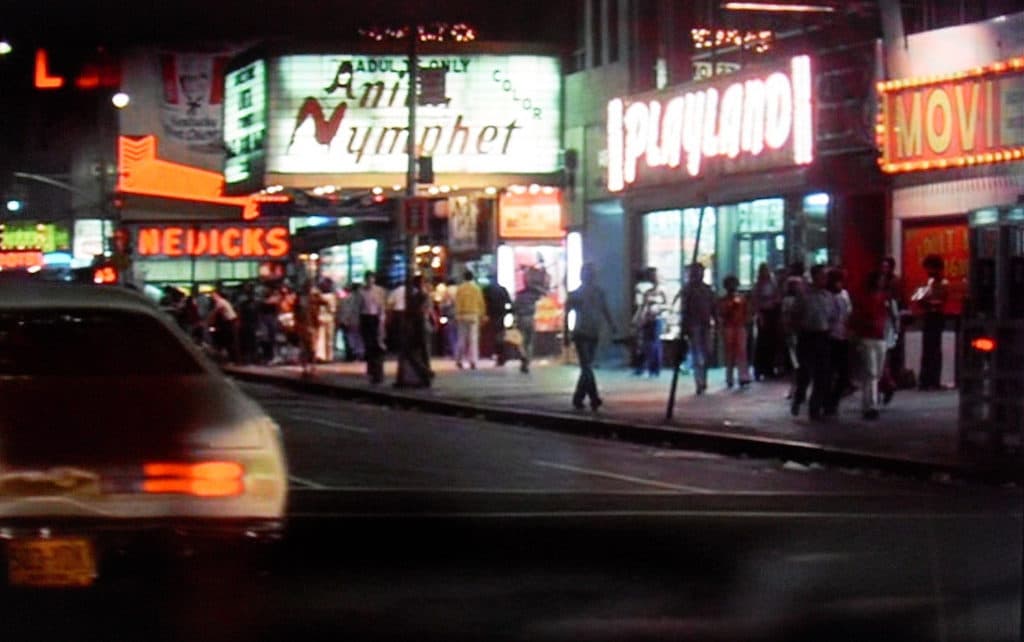
In-brief: The ‘bad neighborhoods’ effect applies to bots, too, according to the firm Recorded Future, which says that it can identify computers that are likely to be involved in botnets, even before they are, based on their neighborhood.
It turns out that the “bad neighborhoods” theory applies to computers, as well as people.
Researchers from the firm Recorded Future said on Thursday that they have developed a way of identifying which systems on the Internet will participate in criminal networks, like botnets, well before they actually become embroiled in such activity. The key, they say, is what online neighborhood those systems inhabit.
Analysis of behavior by systems used to command criminal ‘botnets,’ or networks of infected computers, shows that Internet connected systems located in bad neighborhoods – close to other systems engaged in criminal activity are more likely to become involved in that activity also, Staffan Truvé, CTO, Recorded Future told The Security Ledger.
In a blog post on Thursday, the company said that it applied artificial intelligence (AI) it has developed to identify “future malicious activity” – a kind of ‘future crimes’ report that can predict what computers are likely to be involved in botnets, even before they are.
The technique uses what Recorded Future described as a “support vector machine” (or SVM) model to analyze contextual open source intelligence (OSINT) data on malicious online behavior. That is cross referenced to “CIDR neighborhoods” – blocks of Internet addresses identified using Classless Internet Domain Routing (or CIDR), a method for allocating blocks of numeric Internet Protocol (or IP) addresses to Internet Service Providers and others.
The output is a predictive risk score for specific IP addresses that can alert security operations center (SOC) operators or threat analysts. The score might even be used to automatically block traffic from the address in firewalls and other network security systems, Recorded Future said.
So far the results are promising. Recorded Future said that, in one case, it was able to flag an IP address on October 4 that didn’t actually begin engaging in malicious activity until 10 days later, on October 14, as part of a command and control network for the DarkComet RAT (remote access trojan), a common cyber criminal tool. In general, its AI is identifying future-malicious computers three to five days in advance of them appearing on open source threat lists, the company said.
In an analysis of 500 previously unseen IPs with a predictive risk scores that suggested they would become malicious, 25% turned up on independent, open source lists of malicious IP addresses within 7 days, the company said. By comparison, just %.02 percent of the entire population of global (IPV4) IP addresses are marked as malicious at any time, the company said.
As for why, the explanation that Recorded Future gives sounds similar to the findings of sociological and psychologic research on the effects of bad neighborhoods. The notion there is that “bad neighborhoods” – characterized by crime, poverty and a scarcity of good role models and economic opportunities – can affect the cognitive development of children and even of the children of those children.
In the case of Internet connected systems that are destined to ‘go bad,’ the issue is less economic activity or role models than proximity to computers that are involved in malicious activity, said Truvé. Hackers and botnet operators are rational, economic beings, he observes. That means that they will eventually use infrastructure that they rent for a purpose (like virtual systems in a data center that might be rented out for use in a denial of service attack. By analyzing the “closeness” of IPV4 addresses, Recorded Future found a predictor of future malicious activity.
That was especially true of so-called command and control nodes, which are used to manage massive networks of infected systems. Such systems are far more likely to shift from address to address to avoid detection and so-called “take downs,” like the recent takedown of the Avalanche botnet.
[Interested in machine learning and AI in security? Check out this article, too! ]
All that shifting around is important, Recorded Future found. “If an IP address acts as a (command and control) server, its contagiousness (sp) is different from if it is just being used as a distribution point for malware,” Truvé said. Proximity to one of those bad apples makes it more likely that you’re a bad apple, also – or soon will be, he said. “There’s an underlying logic, which is that the neighborhood (the system) is in will be the core part of whether it becomes malicious, but also how your neighbors are talked about.”
The model isn’t perfect. Truvé said it benefits from lots of high quality historical data to train on. For that reason, the algorithm is more applicable to older IPV4 addresses than the massive IPV6 address space, though Truvé said there’s no reason it can’t work on the latter, as well.
As this blog has noted, machine learning and computer automation is replacing low-level tasks that have been performed by humans. Experts agree that computer automation, powered by machine learning, could soon replace much of the low-level, or “Tier 1” computer security work, like helping users who have been locked out of their account or escalating certain kinds of alerts generated by network monitoring and security tools like antivirus and intrusion detection sensors.
Truve said that Recorded future is hoping the predictive intelligence will allow its customers to get ahead of emerging threats. “Our general idea is that open source (intelligence) is the only way to get a heads up, otherwise you’re fighting hacks that have already happened or that are under way.